Portfolio module 2 {Get Started with Python} Google Advanced Data Analytics Certificate
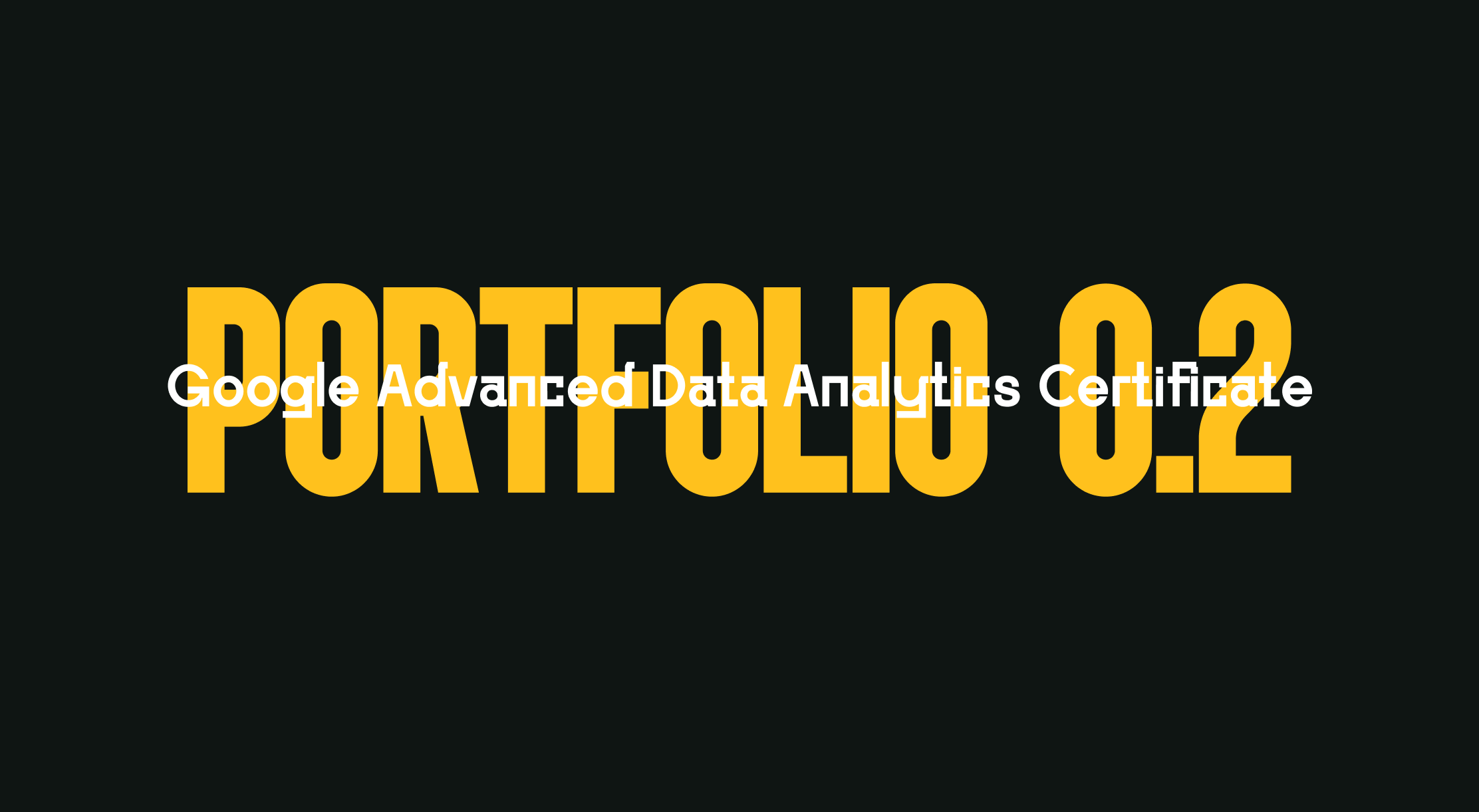
At the beginning there are sc emails from managers with a request. Then my preparation for the PACE strategy document. Then questions from the laboratory and my answers to them. Then the lines of code that were necessary to get answers to the questions. And at the end, a summary for the managers and a report.
Scenario
The team’s latest project is in its early stages of developing a machine learning model to classify claims in videos.
Previously, you were asked to complete a project proposal by your supervisor, Rosie Mae Bradshaw. You have received notice that the project proposal submitted by the team has been approved and your team has been given access to TikTok’s user data. To get clear insights, the data must be inspected, organized, and prepared for analysis.
You discover two new emails in your inbox: one from your supervisor, Rosie Mae Bradshaw, and one from Willow Jaffey, the data team’s Data Science Lead. Review the emails, then follow the provided instructions to complete the PACE strategy document, the code notebook, and the executive summary.
Note: Team member names used in this workplace scenario are fictional and are not representative of TikTok.
Email from Rosie Mae Bradshaw, Data Science Manager
Subject: Help with coding notebook?
From: “Bradshaw, Rosie Mae” —rosiemaebradshaw@tiktok
Cc: “Rainier, Orion”—orionrainier@tiktok
Good morning,
I have a couple of updates on our latest project. The leadership team has approved the project proposal that we completed previously. Thanks for all of your great work so far. Additionally, I just received an email from our Project Management Officer, Mary Joanna Rodgers that the data team is clear to proceed.
Before we begin the process of Exploratory Data Analysis (EDA), we could really use your help with coding and prepping the data. During your interview you mentioned that you worked with Python specifically in the Google certificate program you completed. That experience sounds applicable here.
Orion Rainier (Cc’d above) started a Jupyter notebook with the relevant dataset (attached). Orion is very involved in the final stages of another project. I’m sure your assistance in completing the coding and setting up the notebook for the project would be greatly appreciated.
Orion, do you mind sharing the details?
Humblest regards,
Rosie Mae Bradshaw
Data Science Manager
TikTok
Email from Orion Rainier, Data Scientist
Subject: RE: ****Help with coding notebook?
From: “Rainier, Orion”—orionrainier@tiktok
Cc: “Bradshaw, Rosie Mae” —rosiemaebradshaw@tiktok
Nice to meet you (virtually)!
Hope you have enjoyed your first few weeks!
With the project proposal approved, we are ready to begin the process of preparing the claim classification data. The goal of this project is to ultimately build a machine learning model that can streamline the claims process by identifying whether statements made in videos are claims or opinions.
A claim refers to information that is either unsourced or from an unverified source. For example, “The news reported that someone revealed that around 50% of the mined gold on Earth comes from one source.”
Opinions refer to the personal beliefs or thoughts of a group or an individual. Here’s an example, “In my opinion the most productive work day of the week is Tuesday.”
There are a number of data team members committed to adjusting the machine learning developed for the last project, so your help is greatly appreciated!
Until we finish the prior project, there is no need to do a full EDA on this data. We will get to that soon. Do you mind importing the data (attached) and reviewing it for the team? It would be fantastic if you could include a summary of the column Data types, data value nonnull counts, relevant and irrelevant columns, along with anything else code related you think is worth sharing/showing in the notebook? You’ll need to select a couple of variables to focus on. Include their minimum and maximum values. I haven’t looked closely at the data yet, but it would be really helpful if you can create meaningful variables by combining or modifying the structures given.
Thanks,
Orion Rainier
Data Scientist
TikTok
–
“Big data isn’t about bits, it’s about talent.” — Douglas Merrill
PACE strategy document {Course 2}
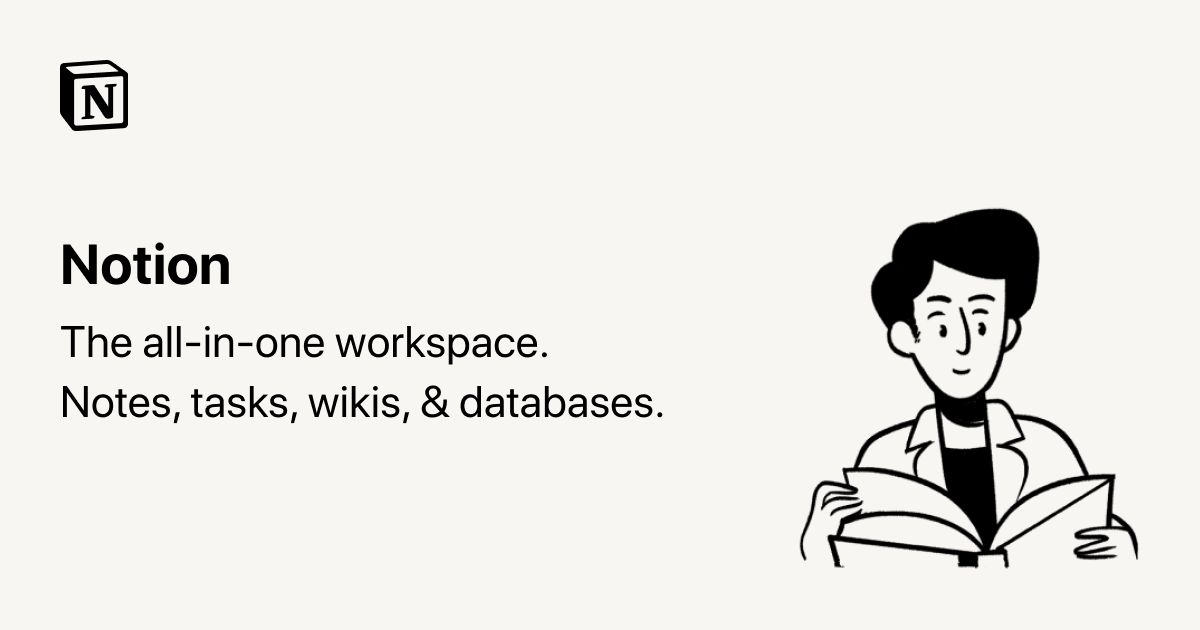
Project lab _Questions
How can you best prepare to understand and organize the provided information?
Begin by exploring your dataset and consider reviewing the Data Dictionary.
When reviewing the first few rows of the dataframe, what do you observe about the data? What does each row represent?
- Row Representation: Each row seems to represent a TikTok video, with various attributes related to the claims made in the video. These attributes include identifiers, content details, verification status, author status, and engagement metrics.
- Observations:
- All videos in the sample are classified as "claim".
- The
verified_status
mainly displays "not verified", indicating that the content's accuracy has not been officially confirmed. - The
author_ban_status
varies, with the majority of authors being "active". This status could affect the perception and reach of their content. - Engagement metrics like view, like, share, download, and comment counts differ significantly among videos, indicating varying levels of user engagement and content popularity.
When reviewing the data.info()
output, what do you notice about the different variables? Are there any null values? Are all of the variables numeric? Does anything else stand out?
- Variable Types: The dataset contains both numeric (e.g.,
video_id
,video_duration_sec
,video_view_count
) and categorical data (e.g.,claim_status
,verified_status
,author_ban_status
). - Null Values: Typically, this stage is where you'd check for null values. In the data snapshot provided, no nulls are visible in these rows, but you'd need to inspect the entire dataset to be sure.
- Standout Features: Non-numeric data like
claim_status
,verified_status
, andauthor_ban_status
will need to be encoded for use in machine learning models. The varied statuses of verification and author ban could potentially affect the interpretation of engagement metrics.
When reviewing the data.describe()
output, what do you notice about the distributions of each variable? Are there any questionable values? Does it seem that there are outlier values?
- Distributions: From the table analysis:
- Video Duration: Most videos are short, typically under a minute, which is common for TikTok content. This length could limit the amount of information conveyed and thus influence claim verification.
- Engagement Metrics: There's a wide range in engagement metrics (views, likes, shares, downloads, comments), with some videos showing exceptionally high numbers, suggesting viral content or outliers.
- Outliers: Some videos, like the one in row 7, have significantly fewer likes compared to views. This discrepancy might indicate either a data error or specific content reactions that warrant further investigation.
- Questionable Values: The significant differences in engagement metrics (e.g., a video with many shares but relatively few views) should be examined for data integrity or unique content characteristics.
What do you notice about the values shown?
The notable aspect of these values is their even distribution between the two claim statuses. The equilibrium between the number of videos labeled as “claim” and “opinion” could be a significant consideration when creating a machine learning model for classifying videos by their claim status. This implies an equal representation of both types of content in the dataset, potentially leading to a more robust and balanced model.
What do you notice about the mean and media within each claim category?
Huge variation in values between ‘claim’ status and ‘opinion’ status.
What do you notice about the number of claims videos with banned authors? Why might this relationship occur?
Based on the provided counts, it’s noticeable that the number of claim videos with banned authors is substantially lower compared to claim videos with active or under review authors.
- Group Data by Author Ban Status: Segment the data based on the
author_ban_status
column. This helps us analyze the difference in share counts between banned and active authors. - Calculate Summary Statistics for Each Group: Compute summary statistics (mean, median, standard deviation) for the
video_share_count
for each group. This gives insights into the share counts' central tendency and dispersion among different author statuses. - Visualize the Data: Use box plots or histograms to compare the share counts' distribution between groups. This aids in visually identifying any outliers or significant distribution differences.
- Statistical Testing: If needed, perform statistical tests like t-tests or Mann-Whitney U tests. These can determine if the share count differences between banned and active authors are statistically significant.
What do you notice about the number of views, likes, and shares for banned authors compared to active authors?
- Views: Both active authors and those under review can attain high view counts. However, active authors tend to have a wider range of views. This suggests that being active may correlate with both moderately and highly viewed videos.
- Likes and Shares: Active authors, especially those with engaging videos (either controversial or highly engaging content), often have high likes and shares. Nevertheless, the author under review with 62,303 shares demonstrates that even potentially controversial or high-interest videos from these authors can garner substantial engagement.
- Engagement Variability: There is considerable variability within each group. This suggests that other factors, such as video content quality, release timing, and topic relevance, significantly influence engagement metrics.
How does the data for claim videos and opinion videos compare or differ? Consider views, comments, likes, and shares?
Likes per View: On average, claim videos receive more likes per view than opinion videos across all author ban statuses. Banned authors’ videos, both claim and opinion, tend to have a slightly higher average likes per view compared to those of active or under-review authors.
Comments per View: Claim videos generally receive more comments per view than opinion videos for all author ban statuses. However, the difference in the average number of comments per view between claim and opinion videos is relatively small.
Shares per View: Claim videos typically have a higher average shares per view than opinion videos, regardless of the author’s ban status. The difference in average shares per view between claim and opinion videos remains consistent across different author ban statuses.
Blocks of code
# What are the different values for claim status and how many of each are in the data?
### YOUR CODE HERE ###
claim_status_counts = data['claim_status'].value_counts()
print(claim_status_counts)
# What is the average view count of videos with "claim" status?
### YOUR CODE HERE ###
claim_videos = data[data['claim_status'] == 'claim']
average_view_count_claim = claim_videos['video_view_count'].mean()
print("The average view count of videos with 'claim' status is:", average_view_count_claim)
# What is the average view count of videos with "opinion" status?
### YOUR CODE HERE ###
opinion_videos = data[data['claim_status'] == 'opinion']
average_view_count_opinion = opinion_videos['video_view_count'].mean()
print("The average view count of videos with 'opinion' status is:", average_view_count_opinion)
# Get counts for each group combination of claim status and author ban status
### YOUR CODE HERE ###
grouped_counts = data.groupby(['claim_status', 'author_ban_status']).size()
print(grouped_counts)
### YOUR CODE HERE ###
median_share_count_by_ban_status = data.groupby('author_ban_status')['video_share_count'].median()
print("Median Video Share Count by Author Ban Status:")
print(median_share_count_by_ban_status)
# What's the median video share count of each author ban status?
### YOUR CODE HERE ###
data.groupby(['author_ban_status']).median(numeric_only=True)[
['video_share_count']]
### YOUR CODE HERE ###
columns_to_agg = {
'video_view_count': ['count', 'mean', 'median'],
'video_like_count': ['count', 'mean', 'median'],
'video_share_count': ['count', 'mean', 'median']
}
author_ban_status_stats = data.groupby('author_ban_status').agg(columns_to_agg)
print("Engagement Statistics by Author Ban Status:")
print(author_ban_status_stats)
### YOUR CODE HERE ###
columns_to_agg = {
'likes_per_view': ['count', 'mean', 'median'],
'comments_per_view': ['count', 'mean', 'median'],
'shares_per_view': ['count', 'mean', 'median']
}
engagement_stats = data.groupby(['claim_status', 'author_ban_status']).agg(columns_to_agg)
print("Engagement Statistics by Claim Status and Author Ban Status:")
print(engagement_stats)
Summary for Rosie Mae Bradshaw and the TikTok Data Team:
- What percentage of the data is comprised of claims and what percentage is comprised of opinions?
- What factors correlate with a video’s claim status?
- What factors correlate with a video’s engagement level?
Following a thorough analysis of the provided TikTok data, the key findings are as follows:
Percentage of Claims and Opinions: The data consists of roughly 49.6% claim videos and 48.9% opinion videos, suggesting a relatively even distribution between the two types of content.
Factors Correlating with Video’s Claim Status: Several factors, including the author’s ban status, video duration, and video transcription content, were found to correlate with a video’s claim status:
Author Ban Status: The distribution of claim status varies based on whether the author is active, banned, or under review. However, this correlation’s extent needs further analysis.
Video Duration: Longer videos are more likely to be classified as claims, indicating that in-depth content may often make factual assertions.
Video Transcription Text: The video’s transcription content may influence its claim status, but specific patterns need further investigation.
Factors Correlating with Engagement Level: Engagement level, measured by likes, comments, and shares per view, correlates with the author’s ban status, claim status, and video content:
Author Ban Status: Videos from banned authors have slightly higher engagement levels per view than those from active or under review authors.
Claim Status: Compared to opinion videos, claim videos generally receive higher engagement levels per view across all author ban statuses. This trend suggests that claim videos may incite more viewer interaction and discussion.
Video Content: The video content, as indicated by the transcription text, may also influence engagement levels. However, specific trends need further analysis.
In conclusion, these findings provide valuable insights into the distribution of claim and opinion videos and the factors influencing video claim status and engagement levels on TikTok. Further analysis will be beneficial to refine these insights and guide decision-making processes for content moderation and platform optimization.
Executive Summary {Milestone 2}
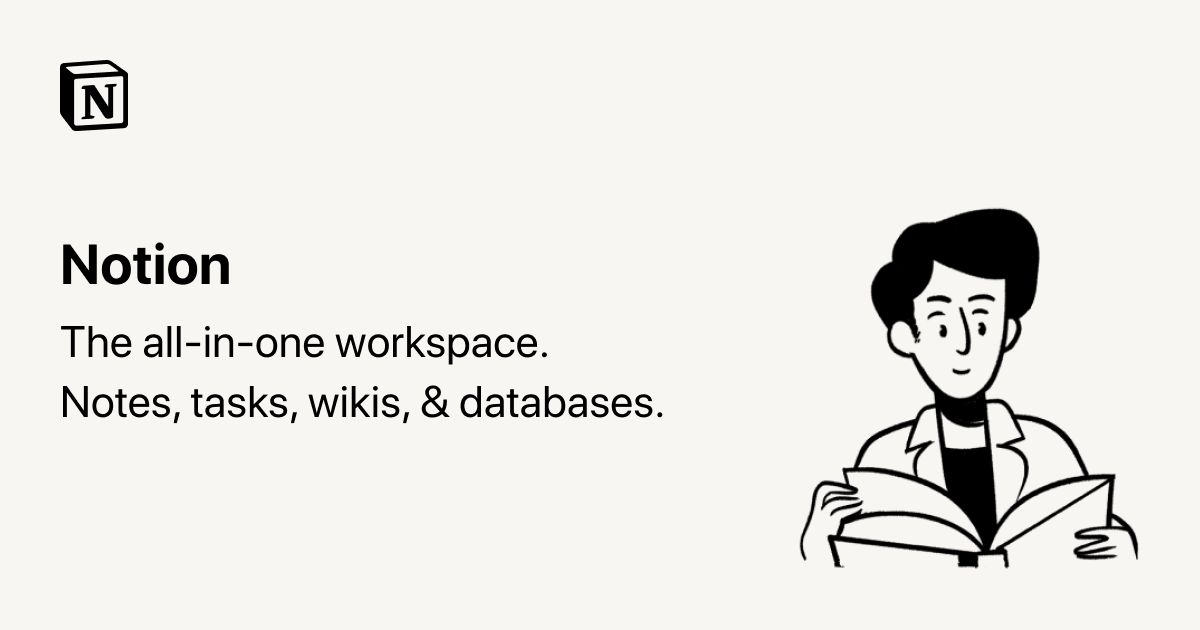